As cyber threats become increasingly sophisticated, traditional security measures are no longer enough to safeguard sensitive data and systems. Enter artificial intelligence (AI) and, more specifically, machine learning (ML). These technologies are transforming the cybersecurity landscape by enabling proactive threat detection and rapid response. In this post, we'll explore how machine learning is revolutionizing threat detection in cybersecurity.
The Role of Machine Learning in Cybersecurity
Machine learning algorithms analyze vast amounts of data to identify patterns, anomalies, and potential threats that may go unnoticed by conventional systems. Unlike rule-based systems, ML can adapt and evolve as new threats emerge, offering a dynamic approach to security.
How Machine Learning Detects Threats
1. Anomaly Detection
ML models learn the typical behavior of networks, systems, and user activities. When they detect deviations from the norm—such as unusual login patterns or data transfers, they trigger alerts. This approach helps in early detection of:
Insider Threats R
Security Concern | Meaning |
Insider Threats | Risks posed by trusted insiders misusing access to compromise data. |
Data Exfiltration | Unauthorized removal or transfer of sensitive data from an organization's system. |
Unusual System Behavior | Deviations from normal operations that may indicate a security breach. |
2. Behavioral Analysis
By analyzing historical data, ML algorithms build profiles of normal behavior for users and devices. Any deviation, such as accessing sensitive information at odd hours or from unusual locations, is flagged as potentially malicious.
Example: If an employee typically accesses internal databases during regular business hours from the corporate office in New York, the system learns that this is standard behaviour. However, if the same employee's account suddenly logs in at 2 AM from an IP address based in Eastern Europe and attempts to access sensitive financial records, the algorithm will flag this activity as a deviation from the norm and potentially malicious. This early detection allows security teams to investigate and respond quickly to prevent any potential security breach.
3. Threat Intelligence Integration
Machine learning systems integrate threat intelligence from various sources to continuously update their databases with the latest indicators of compromise (IOCs). This enables:
• Rapid identification of known malware signatures.
• Detection of emerging threats before they cause widespread damage.
• Swift detection of zero-day vulnerabilities by analyzing abnormal behaviors.
• Prioritization of security incidents based on potential impact.
• Automated alerts and response measures to mitigate threats quickly.
• Continuous refinement of detection models to minimize false positives.
• Seamless integration with other security tools for comprehensive threat management.
4. Predictive Analytics
ML uses predictive analytics to forecast potential threats based on current trends and historical patterns. This proactive approach helps organizations:
• Strengthen defenses before an attack occurs.
• Allocate resources more effectively.
• Enhance incident response by anticipating likely attack vectors.
• Prioritize critical system updates and patches.
• Optimize security budgets with targeted investments.
• Improve risk management through early vulnerability detection.
5. Real-Time Monitoring
Machine learning tools can process and analyse data in real time, enabling immediate detection and response. This is particularly crucial for:
• Distributed Denial of Service (DDoS) attacks
• Ransomware outbreaks
• Zero-day exploits
• Advanced Persistent Threats (APTs)
• Insider breaches
• Phishing attacks
• Unusual network traffic spikes
Benefits of Using Machine Learning in Cybersecurity
Enhanced Accuracy: ML algorithms reduce false positives by learning what constitutes normal behavior.
Scalability: These systems can handle large volumes of data, making them suitable for enterprise-level security.
Adaptability: Machine learning models continuously improve with new data, staying ahead of evolving threats.
Efficiency: Automated threat detection reduces the response time, allowing cybersecurity
teams to focus on strategic tasks.
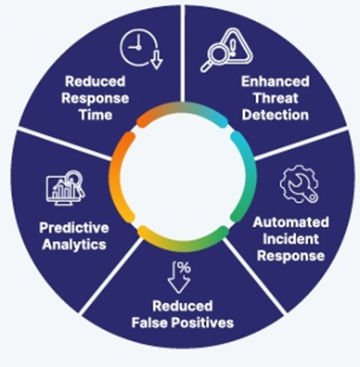
Challenges and Considerations
While machine learning offers significant advantages, it also comes with challenges:
Data Quality: The accuracy of ML models depends on the quality and quantity of the data they are trained on.
Complexity: Implementing and managing ML-based cybersecurity systems require specialized expertise.
Adversarial Attacks: Cybercriminals may attempt to deceive ML models by feeding them manipulated data.
Challenges of AI in Cybersecurity
Final Thoughts
Machine learning is proving to be a game-changer in the field of cybersecurity. By leveraging advanced algorithms for anomaly detection, behavioral analysis, and predictive analytics, organizations can detect threats in real time and respond more effectively. As cyber threats continue to evolve, the integration of AI and ML into cybersecurity strategies will be essential in maintaining robust defenses.
Embracing these technologies not only enhances security but also enables businesses to focus on innovation, confident that their data and systems are well-protected.
Check out our Blog to learn more about Cybersecurity: Importance of Cybersecurity
Follow our LinkedIn page Traceroute Global Services for more insights and updates on Cybersecurity.
#AI #Cybersecurity #MachineLearning #ThreatDetection #AnomalyDetection #BehavioralAnalysis #PredictiveAnalytics #RealTimeMonitoring #DataSecurity #CyberThreats#Whatarethebenefitsand limitationsofusingAIincybersecurity
Comments